Artificial Intelligence vs Machine Learning: What’s the difference?
What do search engines and nature have in common? Both need artificial intelligence (AI) to survive. While it may seem like only one of the two actually needs AI to survive, it is becoming increasingly true for the other to rely on AI to survive as well. Moreover, the use of machine learning (ML) accelerates the process of AI to a level humans cannot possibly undertake. So, what is the difference between AI and ML, and how will it become the cornerstone of objectives like reducing environmental impacts?
What is Artificial Intelligence?
Artificial intelligence is the ability for computers to imitate cognitive human functions such as learning and problem-solving. Through AI, a computer system uses math and logic to simulate the reasoning that people use to learn from new information and make decisions.
Today, artificial intelligence is everywhere. It is used in cell phones, vehicles, social media, video games, banking, and even surveillance. AI is capable of problem-solving, reasoning, adapting, and generalized learning. AI uses speech recognition to facilitate human functions and resolve human curiosity. You can even ask many smartphones nowadays to translate spoken text and it will read it back to you in the new language.
While we are not in the era of strong AI just yet—the point in time when AI exhibits consciousness, intelligence, emotions, and self-awareness—we are getting close to when AI could mimic human behaviors soon.
AI has become an essential function of business development. In fact, customer satisfaction is expected to grow by 25% by 2023 in organizations that use AI and 91.5% of leading businesses invest in AI on an ongoing basis. AI is even being used in oceans and forests to collect data and reduce extinction. It is evident that artificial intelligence is not only here to stay, but it is only getting better and better.
What is Machine Learning?
Machine learning is when we teach computers to extract patterns from collected data and apply them to new tasks that they may not have completed before.
Take a music streaming platform, for example. Certainly, you or someone you know has searched for an artist on a music streaming platform, have found the artist, and have been recommended to a similar artist after scrolling down a bit. Shockingly, whether you know the recommended artist or not, you will notice similarities after listening to them.
Machine learning is a complex and hyper-intelligent process that continuously learns from extracted data—in the case of music streaming platforms, ML can recommend custom songs and artists to you by looking at what other users with similar tastes have listened to.
Artificial Intelligence vs Machine Learning
The relationship between AI and ML is more interconnected instead of one vs the other. While they are not the same, machine learning is considered a subset of AI. They both work together to make computers smarter and more effective at producing solutions.
AI uses machine learning in addition to other techniques. Additionally, machine learning studies patterns in data which data scientists later use to improve AI. The combination of AI and ML includes benefits such as obtaining more sources of data input, increased operational efficiency, and better, faster decision-making.
AI and ML are beneficial to a vast array of companies in many industries. Retail, banking and finance, healthcare, sales and marketing, cybersecurity, customer service, transportation, and manufacturing use artificial intelligence and machine learning to increase profitability, work processes, and customer satisfaction. Additionally, ML can predict many natural disasters, like hurricanes, earthquakes, and flash floods, as well as any human-made disasters, including oil spills.
Real-world AI and ML Application
How do we take machine learning and AI and use them to help save Earth—particularly nature? That is where science and creativity come to play. Humans must be able to interpret the collected data from AI and ML to make decisions and find solutions to world problems. Here are some steps we can take:
1. Comprehend data
In a hyper-connected world, we are surrounded by data. As it gets harder every day to understand the information we are receiving, our first step is learning to gather relevant data and—more importantly—to understand it. Being able to comprehend data collected by AI and ML is crucial to reducing environmental impacts.
2. Make predictions
You can make predictions through supervised learning and data classification. Neural networks in machine learning—or a series of algorithms that endeavors to recognize underlying relationships in a set of data— facilitate this process. Making educated guesses using collected data can contribute to a more sustainable planet.
3. Make informed decisions
You can make effective decisions by eliminating spaces of uncertainty and arbitrariness through data analysis derived from AI and ML. Making informed decisions is different from making guesses. The decision is backed by trusted data from AI.
4. Deploy casual interference
You can infer relevant conclusions to drive strategy by correctly applying and evaluating observed experiences using machine learning.
AI and ML are essential to not only combatting climate change, but also to helping most industries achieve their goals and obtain success.
Machine Learning: From Data to Decisions at MIT Professional Education
At MIT Professional Education, we strive to help professionals achieve success. Our Machine Learning: From Data to Decisions course supplies you with the essential skills to apply AI and ML outcomes to your organization. With the collected data, you can contribute to decision-making aspects in practically any industry.
The instructor of the course, Prof. Devavrat Shah, instructs Electrical Engineering and Computer Science at MIT. He is the founding director of Statistics and Data Science Center, Institute for Data, Systems and Society. His current research interest is in developing large-scale machine learning algorithms for unstructured data with particular interest in social data.
With his guidance, you can learn data comprehension, how to make predictions, how to make better-informed decisions, and how to use casual inference to your advantage. With our machine learning course, you will reduce spaces of uncertainty and arbitrariness through automatic learning and provide organizations and professionals the security needed to make impactful decisions.
Find out more about MIT Professional Education’s courses.
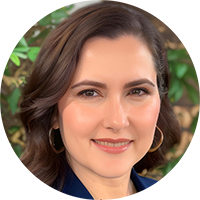
Clara Piloto
Director of Global Programs, Director of Digital Plus Programs
MIT Professional Education
Massachusetts Institute of Technology
professional.mit.edu